Leveraging Big Data
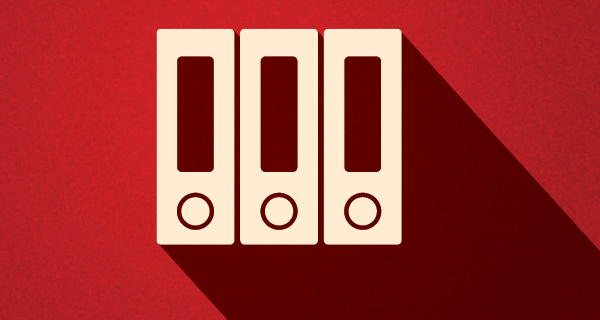
Think big – but start small – when mining big data to propel your supply chain forward.
Big data is big news. In 2013, 64 percent of respondents to a Gartner survey of 720 companies worldwide were investing or planning to invest in big data technologies.
While it is generating interest, the concept of big data also creates a fair amount of confusion. For all their desire to mine insight from the mass of information their companies collect, many corporate leaders are puzzled about how to put big data to work. Among the respondents to the Gartner survey, 56 percent say determining how to get value from big data is a major challenge, and 41 percent cite challenges with defining their big data strategies.
What’s the big deal about big data? How can companies use it to better manage logistics and supply chain operations?
First, a few definitions.
Big data usually means the practice of collecting electronic information from numerous sources and applying analytics to identify patterns, trends, and other intelligence. The analysis might point to things that have happened but weren’t easy to perceive, or it might help a company predict what will happen in the future.
One company using big data in supply chain management is Amazon, which recently filed a patent for technology to support “anticipatory shipping.” This technique will help the online retailer adjust inventory to anticipate customer demand in specific locations, based on factors such as previous purchases, which products customers searched for online, and how long they spent looking at specific items.
“Amazon wants to pre-position items based on understanding its customers and their demographics, identifying trends, and ramping up to match them,” explains Bret Wagner, associate professor of management and director of the integrated supply chain management program at Western Michigan University (WMU), Kalamazoo, Mich.
The “big” part of big data refers to the volume of information available to analyze. In the supply chain realm, that might include data from point-of-sale systems, bar-code scanners, radio frequency identification readers, global positioning system devices on vehicles and in cell phones, and software systems used to manage transportation, warehousing, and other operations.
Numbers, Text, and More
But volume is just one of several factors that define big data. A second is variety. Not only does the data come from a wide range of sources, but it may include more than structured data—information recorded in fields within databases. Big data may also encompass information contained in text, image files, and other formats.
“The data is not just numbers anymore,” says Erick Brethenoux, director of business analytics and decision management strategy at IBM. “Companies have access to a lot of unstructured data—for example, from social media sites, online communities, and call centers. They also get feedback from drivers about vehicle performance.”
Tweets, “likes,” blog posts, emails, product reviews, and online comments all hold information that, when aggregated and analyzed, can help companies determine what customers want, and when and where they’ll want it.
A third dimension of big data is speed. “It used to be sufficient to review models or analyze data daily, weekly, or monthly,” says Brethenoux. But today, if companies want to head off problems such as inventory shortfalls, or late deliveries due to bad weather, they must conduct analyses in real or near-real time.
“The importance of speed has accelerated tremendously in the past 10 years, because customer service expectations have changed radically,” he says.
Extracting Insight from Oceans of Data
Although the term big data is a recent addition to the lexicon, the idea that companies might pull important insights from vast oceans of ones and zeroes is not new.
“There have always been cases where data has been too big for the available tools,” says Mark Flaherty, chief marketing officer at InetSoft Technology, a business intelligence technology developer based in Piscataway, N.J. In the 1980s, for example, telecommunications providers collected data on the traffic they carried, in volumes too large for them to analyze through available means.
Today, however, the market offers new tools for extracting meaning from masses of data, so big data is garnering fresh attention.
“The influence of the Internet, global commerce, and analytics technologies to respond to constantly changing world and market conditions drives companies to look for new ways to be competitive,” says Mary Shacklett, president of Transworld Data, a technology and supply chain consulting and research firm in Olympia, Wash. Companies harness big data technology in the hope of gaining innovative knowledge that provides new insights and strategies, she says.
One technology that has emerged to provide those insights is in-memory computing.
In the past, companies that wanted to analyze data from transactions in enterprise resource planning (ERP) and other operational systems would move that data into separate business warehouses. Those repositories resided on high-capacity hard drive systems, so they could handle a lot of data.
“But the speed was slow,” Wagner notes. It was also hard to ensure that the data being analyzed was up to date and accurate.
Today, it is possible to conduct big data analysis within an ERP system while it runs transactions. “With business warehouse systems, the data could be a few days old,” Wagner says. “Now users can query right to the second—for example, locating materials, trucks, or planes—without disrupting the system’s operation.”
The market also offers tools that handle data in numerous formats. “Technologies allow companies to leverage and quickly combine structured, unstructured, and semi-structured data at such a rapid pace that data is available in a central repository that doesn’t require the traditional design that enterprise data warehouses require,” says Steffin Harris, North American big data lead at Paris-based technology consulting firm Capgemini.
Some tools lay one kind of data over another—for example, inbound shipment data onto weather forecasts for the regions those shipments will traverse—to support predictions.
In addition, developers offer visualization tools that pour the results of data analyses into reports and on-screen dashboards for easier interpretation.
Digging into 5 Million Transactions
Among supply chain organizations, much of the big data action involves mining information from large volumes of business transactions. Shippers use business intelligence tools to pull data from multiple sources, merge and analyze it, and create reports to present the results.
One company that uses big data that way is Avnet, a Phoenix-based global distributor of electronic components. Mainly a small-parcel shipper, Avnet conducts more than five million shipping transactions annually. “Each of those transactions has more than 50 columns of data, resulting in more than 250 million data values,” says Marianne McDonald, the company’s vice president of global transportation.
That’s far more data than Avnet can review productively on its own. So the company contracts with a service provider to audit and pay carrier invoices.
The main goal of this partnership is to ensure Avnet pays only what it owes for transportation. But the service provider also gives Avnet tools to pull business intelligence from shipping data.
One report the vendor provides is a series of key performance indicators (KPIs) that identify which carriers make the most invoicing errors. “This type of information enables us to meet quarterly with carriers, and review how their performance stacks up against other carriers we use,” McDonald says. Carriers that don’t improve performance risk losing Avnet’s business.
The tool also reveals how much Avnet’s business units spend on different transportation services, such as next-day, second-day, and three- to five-day ground.
“In the past, it wasn’t possible to identify a unit’s shipping style,” says McDonald. “Now we can pinpoint—to the penny or percentage, and by service level—exactly what those styles look like.” Then her team recommends money-saving adjustments, such as swapping a three-day service for a less-expensive three- to five-day option.
Information about shipping styles also helps Avnet negotiate with carriers more intelligently. “This data helps my team strategize the direction for our next discussion about requests for proposals, because we now understand exactly what we’re shipping,” McDonald says.
More recently, Avnet has started using big data to help decide where to locate distribution hubs around the globe. “That work has progressed from an offline, intensive exercise—putting data from a lot of sources into a spreadsheet—to a more analytics-driven approach, with the tool doing 95 percent of the work,” says Mike Buseman, Avnet’s chief global logistics and operations officer. With technology to crunch the numbers, planning teams can now focus on developing strategy.
Where’s the Greatest Impact?
Lancaster, Ohio-based Glasfloss Industries, which manufactures air filters for heating, ventilation, and air conditioning systems, is also mining its transaction data. In 2009, the company contracted with Hickory, N.C.-based third-party logistics (3PL) provider Transportation Insight to help with carrier selection and routing, and to manage freight claims and audits. In 2013, Glasfloss started using Transportation Insight’s analytics solution, Insight Fusion, to uncover new intelligence about its supply chain.
Insight Fusion merges data from different supply chain systems and outside data sources—such as transportation management, warehouse management, resource planning, and manufacturing—and provides access to a company’s complete supply chain information in real time.
“It doesn’t matter where the data comes from, or the format in which we receive it,” says Jim Taylor, vice president of information technology at Transportation Insight. Technology is now available to translate it all, and manage it in an enterprise data warehouse.
Users access Insight Fusion through a Web portal using a PC, mobile phone, or tablet to display custom-designed reports about their operations.
Information from Insight Fusion will help make significant improvements to the company’s logistics operations, says Greg Gardner, manufacturing operations manager at Glasfloss. “It will allow us to better focus time and effort, and the company’s money, on areas that have the most impact on customer satisfaction or the bottom line,” he explains.
One fact the tool has already revealed is that Glasfloss sends an unusually large number of shipments to one particular state. Thanks to that insight, Glasfloss is now seeking a carrier that offers better rates to that area, based on volume.
The company also is looking at performing consolidation for a few customers, which could help control costs and improve customer service. “If we’re sending three small shipments to the same location for a customer, we’ll suggest consolidating them, even if it means waiting an extra day or two for delivery,” Gardner explains.
Thanks to Insight Fusion, Gardner decided to convert some less-than-truckload (LTL) shipments into two- or three-stop truckloads. Even if Glasfloss pays extra for a multi-stop run, it still costs less to move freight that way than by LTL. “The numbers made that very clear,” he says.
The biggest benefit from Insight Fusion arose when Glasfloss examined customer damage claims by manufacturing plant and by state. “We realized that certain states filed more claims compared to others,” Gardner says. Further investigation showed that by improving the way it ships to just a handful of customers, Glasfloss could dramatically reduce damage claims.
“This is exciting because we will see a significant return on a limited number of transactions,” Gardner says.
To help curb damage, Glasfloss is looking for better ways to ship to those customers. “For example, if we’re shipping loose freight, maybe we need to palletize,” Gardner says. “We also switched carriers in one situation, because we determined it just wasn’t a good lane. And we’re looking at running a two-stop truckload instead of multiple LTLs to another customer.”
Insight Fusion has helped Glasfloss reduce the number of claims filed by 36 percent, and increased its 60-day claims closure rate by 83 percent.
Pinpointing Mispicks
In Cleveland, Hillcrest Foodservice has been using InetSoft’s Style Intelligence tool since 2010. Hillcrest distributes food to restaurants, retail stores, and institutions in northern Ohio and western Pennsylvania.
“The InetSoft system allows more management team members to access information in a quick, standardized manner,” says Jim Schnurr, director of customer solutions at Hillcrest Foodservice.
In the past, managers had to pull data from multiple systems, including a Retalix enterprise solution running on an IBM AS/400, a Roadnet Technologies routing system, and several Microsoft Access databases. Moving those numbers into Microsoft Excel to create presentations was a laborious process.
“The information was there, but I had to rebuild it each time,” recalls Schnurr. Style Intelligence automates that process.
The system also paved the way for several operational improvements. For example, in the past, if a pizza restaurant complained that Hillcrest delivered ketchup instead of tomato sauce on several occasions, getting to the root of the problem would have been hard. To find out whether human error or a system glitch was to blame, Schnurr and his team had to move data from Retalix into Access, and set up a query.
“As wonderful as Access is for grabbing and communicating information, the reporting function is not as user-friendly,” Schnurr says. “With InetSoft, we can run the report automatically.”
Style Intelligence qualifies as a big data solution because, for a company of its size, Hillcrest manages a great deal of data. “We have about six million lines of invoice detail over several years, with 50 fields,” says Schnurr.
The company also captures a bar-code scan each time a warehouse worker picks, pulls, or relocates product. “We need that capability to identify which worker picked an order,” he says. Hillcrest uses the data to manage an incentive program based on picking accuracy.
Hillcrest recently implemented a mobile delivery management system called Driver Pro, from SAE Systems, which has started feeding driver activity data to InetSoft. “We’ll know whether our drivers arrive on time, which is critical for service reporting,” Schnurr says.
The new data also will help determine whether Roadnet is allotting drivers the right amount of time to complete a delivery at each location. “Different products can take different amounts of time to deliver,” he notes. “This new analysis will allow us to make more informed decisions and, we hope, service our customers better.”
Delay the Dye
Consultants who work with companies on analytics projects cite additional supply chain improvements that result from big data projects. Shacklett recalls an online retailer that uses sales data to predict what color sweaters consumers will buy in the greatest quantities at different times of the year. As a result of that information, the company now has its suppliers make sweaters without color, then dye them later, based on customer demand determined in near-real time.
Harris cites a client whose shipments often reached customers late, especially from December through March. The company was losing money as a result, and management wanted to know why. An assessment showed that the company wasn’t dynamically connecting shipping, inventory, or sales information. Nor was it monitoring comments about its product in social media.
The company also wasn’t taking advantage of third-party weather information. “Because no one was able to predict an impact based on weather, they could not make decisions about how to reroute, optimize routes, or pull inventory from another location to meet demand,” says Harris.
With all that data in place, Capgemini ran models that showed the pros and cons of taking different actions in response to weather delays in various situations. “Giving the company the ability to make decisions at just-in-time intervals empowered it to be more effective in managing the operation,” he says.
For companies that want to harness big data in the supply chain, one major challenge might be finding employees with the knowledge to exploit the opportunity.
“Hire people as quickly as you can,” advises Brethenoux. “An analytics skills gap is developing, and if you start looking in one or two years, the talent won’t be available to help you.”
Wagner agrees that it’s hard to find people with the right skills to implement big data. WMU’s integrated supply chain management program is trying to fill that need—for example, by adding a business analyst minor.
In the absence of new hires with specialized qualifications, a company might fill the gap by training people already in its ranks—perhaps in the finance or IT departments—who show a flair for analytics.
It’s also important to educate managers who will integrate the results of analytics into their decision-making. “They have to understand what’s possible—and what’s not possible—with analytics,” Brethenoux says.
If you implement a big data project effectively, valuable new insights and business improvements will follow.
Diving Into Big Data
Whatever you do, don’t wait too long to dive into big data. “Start with a small scope, and do it right away,” recommends Erick Brethenoux, director of business analytics and decision management strategy at IBM.
Mark Flaherty, chief marketing officer at business intelligence technology developer InetSoft Technology, agrees. “Don’t worry about trying to plan out your ultimate business solution from beginning to end,” he says. “Just get started with one burning request from one department.” The experience will provide a valuable education, and prompt others in the company to ask for business intelligence solutions as well.
When you start a project, make sure you’re using good-quality data. “If the data that you pour into your big data analytics process is of low quality, your results won’t be reliable,” says Mary Shacklett, president of technology and supply chain consulting and research firm Transworld Data.
Before starting a big data initiative, develop a clear business case describing how you want to use your data and what you expect to gain from the exercise. “Most importantly, don’t let a vendor define this for you,” Shacklett says. “And if you don’t have big data expertise or resources internally, take the time to research a dependable vendor-partner that can help you.”
Don’t become so dazzled by all the available technology that you fail to define your strategy and goals. “Many times, you can chase the technology and lose the opportunity to solve the business problem in a meaningful way,” says Steffin Harris, North American big data lead at technology consulting firm Capgemini. “Start with the business problem, then the technology will naturally follow.”