AI Has a Black Box Problem, Here’s How to Avoid It
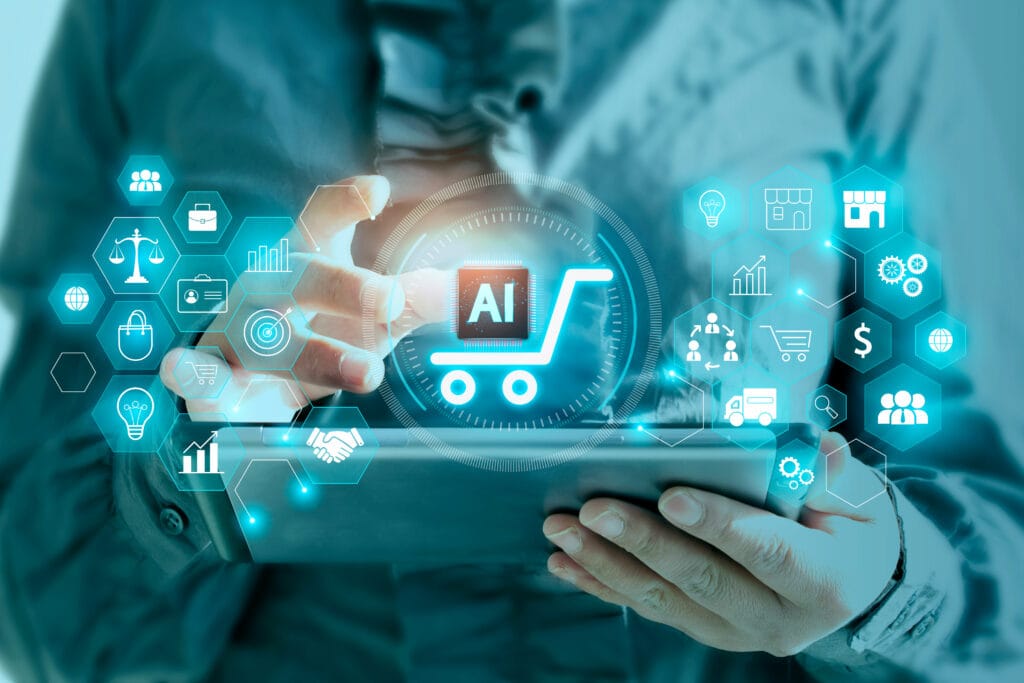
Retailers beware! Before you dive into artificial intelligence solutions to keep your shelves restocked, consider the ramifications of AI’s lack of visibility into how it reaches its output, and whether a slower approach to AI can yield better longer-term outcomes.
It’s hard to put into words just how much ChatGPT has changed our approach to artificial intelligence in such a short period of time—a program that wasn’t even on our radar last December is already poised to upend the way millions of us do our jobs. It seems as if every day there’s a new use case for the tool.
But with every new technology comes reasons for us to be cautious, and for retailers, ChatGPT offers a big one: It doesn’t provide visibility into how it reaches its output. It’s essentially a black box, offering business solutions in seconds without showing its work.
Yes, time is money in the supply chain, and this new technology promises untold efficiency. Before you dive into AI solutions to keep your shelves restocked, however, it’s important to consider the ramifications, and whether a slower approach to AI can yield better longer-term outcomes.
The drawbacks of black box
Let’s say I own a small grocery store, focused mainly on fresh foods. I have 100 oranges, and by close of business, I need to submit an order to my supplier to ensure I have enough oranges to get me through the next two weeks.
I ask a ChatGPT-like program how much to order, and using its algorithm, the tool instantly tells me I need to order an additional 200 oranges. Then I watch 300 oranges sell in three days, leaving my shelves empty, customers upset, and me scrambling to order additional oranges.
Why did the program miss its prediction so spectacularly? There’s no way to tell, and therefore no way to adjust its algorithm for my store specifically, because the data set is a black box. It might not have considered sales trends over time for my town or factored in seasonal impacts. Either way, I can’t correct it, and therefore my trust in the program is irreparably damaged.
The same issue can manifest itself across store operations: predicting the success/failure and potential profit margins of a promotion, building planograms that enable customers to move efficiently through the store, or even answering customer questions online via chatbot. Advanced AI can offer solutions quickly, but the devil is in the details.
Enhancing transparency and trust using human-machine collaboration
To enhance transparency and establish trust in AI-driven retail solutions, human-machine collaboration interfaces can be utilized. Such interfaces enable users to interact with the AI system in real-time and provide continuous feedback, which helps in comprehending the decision-making process.
Employing explainable AI methods such as SHAP or LIME can display the system’s data inputs and can feature importance scores to retailers, thereby increasing their understanding of the system’s recommendations.
A hypothetical example of such an interface is a dashboard that shows real-time sales data and AI-generated stock level suggestions along with feature importance score visualizations using SHAP or LIME. By providing additional information, retailers can adjust or correct the system’s decisions, which in turn enhances their trust and confidence in the system for the long run.
Automate in small doses
ChatGPT and similar black-box programs that handle the thinking for you may be dominating headlines, but employing AI in your store isn’t an all-or-nothing proposition—in fact, finding the right balance of human and AI brainpower is the key to building the automated retail experiences of the future.
For many retailers, especially those just beginning their AI journey, a rules-based solution can be the right option for avoiding black box complexities. Instead of trusting entirely on the AI’s dataset, rules-based solutions allow retailers to feed their own data into the tool and uncover quick solutions grounded in their own sales trends and regional conditions. And in the event the program offers projections that result in a shortage or overage of stock, or an unsuccessful promotion, the retailer can dig through the dataset to figure out what went wrong and how to fix it—there’s no mysterious “man behind the curtain.”
Let AI be your guide—but give it a good map
Data, when used effectively, offers untold benefits to retailers—knowing when to cut back on unpopular items, how to keep fast-selling items on shelves, and strategies for streamlining labor-intensive tasks throughout the store. AI-driven tools help us unlock data’s benefits, but only if we understand the data it’s using, and how it’s using it. Jumping headfirst into AI without this firm understanding will only lead to frustration.
Instead, look at implementing tools that mix control over data with advanced algorithms that truly help you leverage business information, and shine a light into the black box.
In conclusion, while AI systems offer exciting opportunities for retailers to improve their operations, it’s important to approach them with caution. The black box problem, in which the AI’s decision-making process is not transparent, can lead to irreparable damage to trust when errors occur.
Employing human-machine collaboration interfaces and explainable AI methods like SHAP or LIME can help to enhance transparency and build trust in AI-driven retail solutions.
Additionally, retailers can consider a rules-based solution that allows them to feed their own data into the tool and maintain control over decision-making.
Ultimately, the key to successful AI implementation is finding the right balance between human and AI brainpower and understanding the data being used to guide decision-making. With a thoughtful approach, retailers can leverage the benefits of AI to optimize their operations and stay ahead of the curve in the competitive retail landscape.
Here’s a look at 20 AI applications in the supply chain.