ProvisionAi and Riviana Foods: Get a Load of This
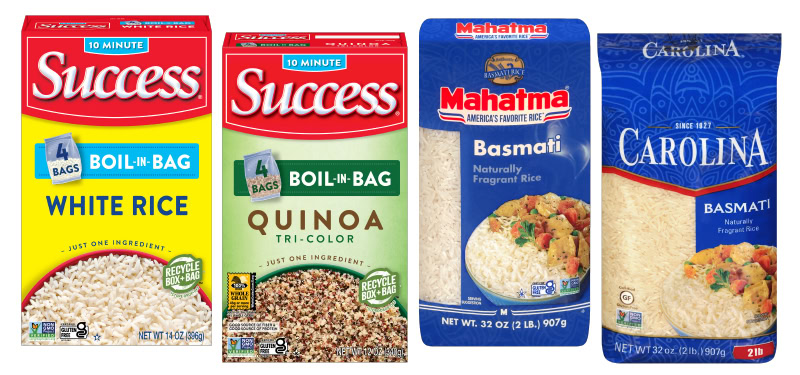
Walk through any grocery store, and you’ll likely come across products from Riviana Foods. It’s one of the largest processors, marketers, and distributors of branded and private-label rice products in the United States. Its brands include Minute, Success, Mahatma, Adolphus, RiceSelect, and others.
THE CUSTOMER
Riviana Foods is a leading rice company and marketer of wild rice. The company is a wholly owned subsidiary of Ebro Foods, which operates in the rice sector across the globe through an extensive network of subsidiaries and brands in more than 80 countries spanning Europe, North America, Asia, and Africa.
THE PROVIDER
Franklin, Tennessee-based ProvisionAi offers sophisticated suites of optimization tools for load building and transportation load leveling that reduce total supply chain costs.
Like many companies over the past few years, Riviana has faced challenges when looking for trucking capacity, especially when demand spikes. One way companies can address this, even when capacity tightens, is to ensure all truckloads are optimized to their maximum legal capacity when they leave a facility.
To help its supply chain organization meet this goal, Riviana turned to Auto02 (Automatic Order Optimization), a load-building solution from ProvisionAi.
Selling a Broad Profile
As a large rice processor—its plant in Memphis, Tennessee moves more than 10,500 shipments annually—Riviana sells a wide range of products to retailers, industrial companies, and food service organizations, among other clients.
Shipments can vary from small, lightweight rice cups to 20-pound bags of rice geared to food service operations. “It’s a broad profile,” says Jennifer Phillips, Riviana’s director of transportation. Shipments generally travel by box car, intermodal, and truckload.
Riviana Foods is a wholly owned subsidiary of Ebro Foods, a global leader in the rice vertical. Ebro Foods’ network of subsidiaries and brands spans more than 80 countries across Europe, North America, Asia, and Africa.
The range of products and weights Riviana ships can present challenges when trying to load a truck so all the space within the trailer is fully utilized. Riviana had been using a load planning solution, but even so, trucks would often ‘weigh out’ before they ‘cubed out’, says Zachary Dale, Riviana’s supply chain continuous improvement manager.
Optimizing Truckloads
In the United States, most truckload shipments travel on 53-foot trailers that provide a bit more than 4,000 square feet of capacity, says Tom Moore, founder and CEO of ProvisionAi.
Most trucks traveling on highways can haul between 45,000 and 50,000 pounds. Because many of Riviana’s products—like sacks of rice—are heavy, shipments often meet the weight capacity of the truck before they’ve actually filled the space available.
The breadth of products Riviana offers and the range of pallet weights can also make it challenging to train new employees to properly load trucks.
“More efficient loading and better truck utilization could cut both the number of trucks on the road, as well as transportation costs,” Phillips says.
Generally, when shipping a variety of product sizes and weights, combining products of varying weights on a truck, rather than filling it with a single product, more efficiently uses the legal weight and space available. Even when dealing with slight differences in weight, there are opportunities to take advantage of that difference in the load design.
A Good Fit
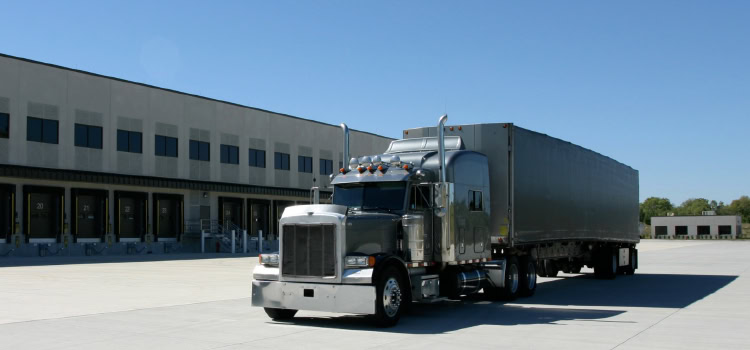
When shipping a range of product sizes and weights, like Riviana does, combining products of varying weights on a truck, rather than filling it with a single product, more efficiently uses the legal weight and space available.
To help optimize truck capacity, Riviana began working with ProvisionAi. As Dale had been researching sustainability, a key initiative at Riviana, he came across information on the AutoO2 solution from ProvisionAi. “It sounded like a good fit and referenced a lot of the issues we were having,” he adds.
Auto02 load building software uses machine learning to optimize shipments, while it also considers more than 300 parameters, including product and customer-specific loading rules, among others.
For instance, a shipper may require that all shipments of a specific product family be placed in the tail of the truck, or that all pallets be shipped on the narrow dimension. The software can accommodate this. It has been deployed by companies around the globe and across verticals.
Along with enabling companies to fit more products on fewer trucks, the Auto02 solution manages the placement of pallets so that lighter weight products are placed on top of heavier ones—“eggs on top of bricks,” as Moore says.
In addition, pallets are placed so that they’re supported when, for instance, a truck must turn sharply. This helps eliminate much of the damage that can occur during transit and from material handling.
AutoO2 bolts on to companies’ enterprise resource planning and warehouse management systems, so it can create and guide large orders through execution. It also creates diagrams workers can follow to guide them as they load shipments onto the trucks. This helps ensure that what is planned actually is loaded, and that the resulting shipments comply with relevant regulations and are protected against most damage.
ROI In Weeks
In some cases, the solution has cut deployment freight expenditure by more than 10%. Many shippers see a return on their investment within a few weeks of going live, the company says.
As Riviana worked to implement the Auto 02 solution, Dale and his team sought input from multiple departments across the organization, including the warehouse, information technology, packaging, and transportation planning teams. This helped ensure all gained a solid understanding of the solution, its potential impact, and how it could help them load more efficiently.
“This helped with the success of the implementation,” Dale says. “Everyone was on the same page and understood the goals.”
Step-By-Step Implementation
The implementation process ran about four months, Dale says. Steps included cleaning the data, and some development in SAP. Among other actions, the teams needed to develop a way to electronically send information, such as the item master with the dimensional and weight data for each product.
In addition, the solution needed to transmit the requirements that the supply planning systems needed to ensure enough inventory would be on hand in customer-facing distribution centers.
For instance, a requirement might say that Location A needs 200 cases of wild rice. Most of the development used standard SAP functionality, Moore says.
Through the connection with Riviana’s supply planning system, Auto02 knows which items are headed to which distribution center. Based on this information, Auto02 can develop truckloads that are both legal and optimized.
When Auto02 went live in late 2022, most operators quickly learned how to use the load diagrams, Dale says. The Auto02 solution reduced the learning curve for new loaders by about 80%, Phillips adds.
Almost as soon as operators can read load patterns, they’re ready to begin loading; they don’t need to first become experts in the products themselves, as well as which are stackable and which aren’t.
Riviana’s supervisors can run the programs to create loads when operators come in each day. If the inventory of loads starts running low during their shift, the supervisors can generate additional plans.
Since implementing Auto02, Riviana has seen about a 3.5% increase in weight per truck. Because freight costs fluctuate, it’s difficult to attribute changes in overall freight expense to the software. However, the increase in weight per truck, “adds up in terms of cost savings,” Dale says.
Suggestions for Improvement
The ProvisionAi team has been flexible and open to suggestions that can improve the solution, Phillips says.
For instance, ProvisionAi helped Riviana streamline the process of importing Excel files for times when demand might veer from what was expected. This could occur when a special promotion is planned that will drive demand in specific locations, and the Riviana operations planning team needs to manually tell the system where to ship products.
Now, the system will still produce a loading diagram, even though the load has been created manually. “They’re helpful in coming up with solutions for any issues or for improving the system,” Dale says.
The ProvisionAi team also has offered suggestions to help Riviana continue to leverage the solution. For instance, the team suggested weighing trucks to understand “weight per load goals.” Because the total legal weight that can be driven on U.S. highways is 80,000 pounds, if a truck weighs 35,000 pounds, as many have, the payload can be 45,000 pounds.
Weighing the Options
Over the past several years, trucks have tended to become lighter, as manufacturers try to increase mileage and allow for higher payloads. However, a loader who is used to working with the heavier trucks of the past might assume a truck weighs more than it actually does.
“By taking the time to weigh each truck, rather than relying on memory, loaders might learn that some are 32,000 pounds,” Moore says. “This means they can increase payload targets to 48,000 pounds.”
Riviana currently has plans to implement Auto02 at its plant in Freeport, Texas, which is the company’s second-largest plant.
Case Study: Taking Up Space
Challenges:
Riviana needed to optimize truckloads to use as much trailer space as possible, cutting costs and number of trucks on the road.
Solution:
Implement Auto02 load optimization software from ProvisionAi.
Results:
An average increase in weight per truck of 3.5% and an 80% reduction in the training time many new loaders require.
Next Steps:
Implement Auto02 in Riviana’s Freeport, Texas plant.